
Artificial Intelligence
Comparative Analysis of Various Machine Learning Techniques for Epileptic Seizures Detection and Prediction Using EEG Data
Epileptic seizures occur as a result of functional brain dysfunction and can affect the health of the patient. Prediction of epileptic seizures before the onset is beneficial for the prevention of seizures through medication. Electroencephalograms (EEG) signals are used to predict epileptic seizures using machine learning techniques and feature extractions. Nevertheless, the pre-processing of EEG signals for noise removal and extraction of features are two significant problems that have an adverse effect on both anticipation time and true positive prediction performance. Considering this, the
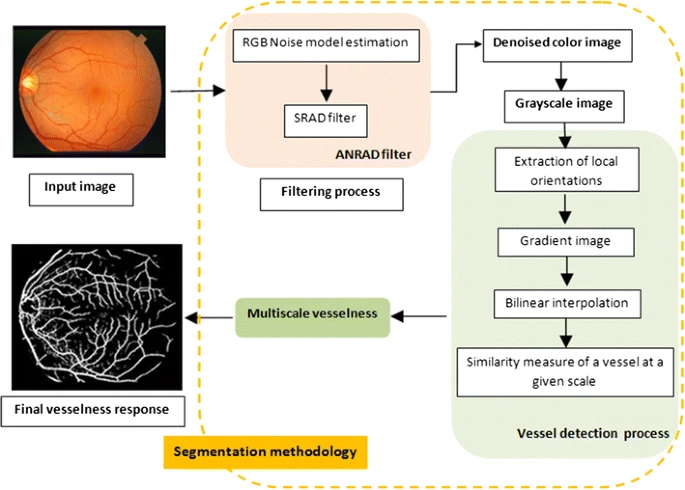
Noise-estimation-based anisotropic diffusion approach for retinal blood vessel segmentation
Recently, numerous research works in retinal-structure analysis have been performed to analyze retinal images for diagnosing and preventing ocular diseases such as diabetic retinopathy, which is the first most common causes of vision loss in the world. In this paper, an algorithm for vessel detection in fundus images is employed. First, a denoising process using the noise-estimation-based anisotropic diffusion technique is applied to restore connected vessel lines in a retinal image and eliminate noisy lines. Next, a multi-scale line-tracking algorithm is implemented to detect all the blood
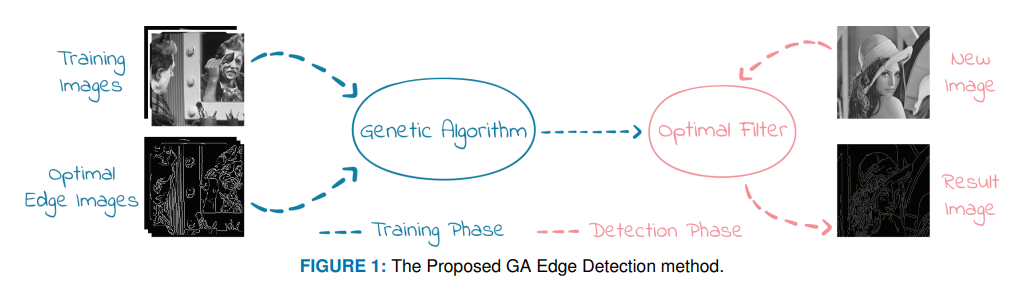
Optimized Edge Detection Technique for Brain Tumor Detection in MR Images
Genetic algorithms (GAs) are intended to look for the optimum solution by eliminating the gene strings with the worst fitness. Hence, this paper proposes an optimized edge detection technique based on a genetic algorithm. A training dataset that consists of simple images and their corresponding optimal edge features is employed to obtain the optimum filter coefficients along with the optimum thresholding algorithm. Qualitative and quantitative performance analyses are investigated based on several well-known metrics. The performance of the proposed genetic algorithm-based cost minimization
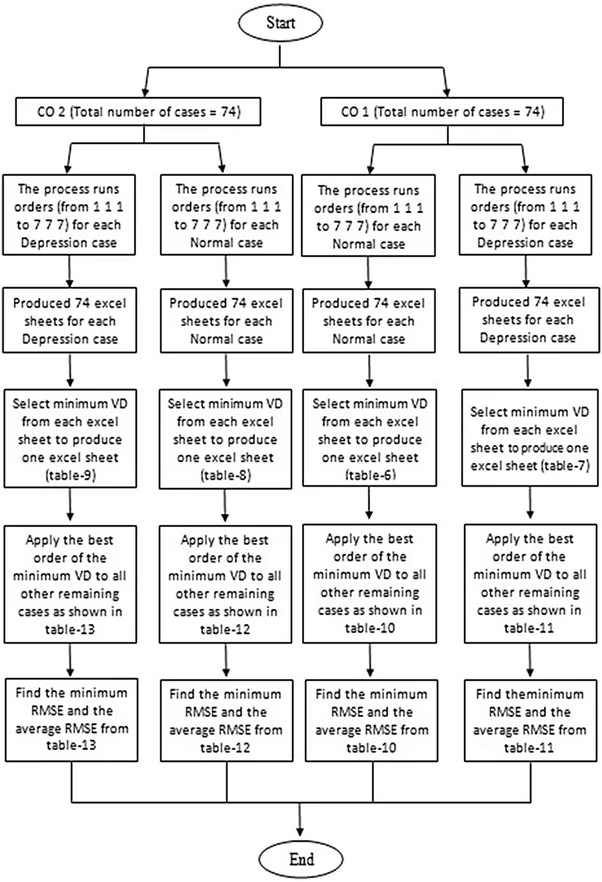
Nonlinear single-input single-output model-based estimation of cardiac output for normal and depressed cases
Mental depression is associated with an increased risk of cardiovascular mortality, thus provisioning generic simple nonlinear mathematical models for normal and depressed cases using only heart rate (HR) or stroke volume (SV) as a single input to produce cardiac output (CO) as a single output instead of using both HR and SV as two inputs. The proposed models could be in the future an effective tool to investigate the effect of neuroleptic medication, especially depression, and it reduces the time of processing. Seventy-four depressed cases, 74 normal peers and autoregressive considered as a
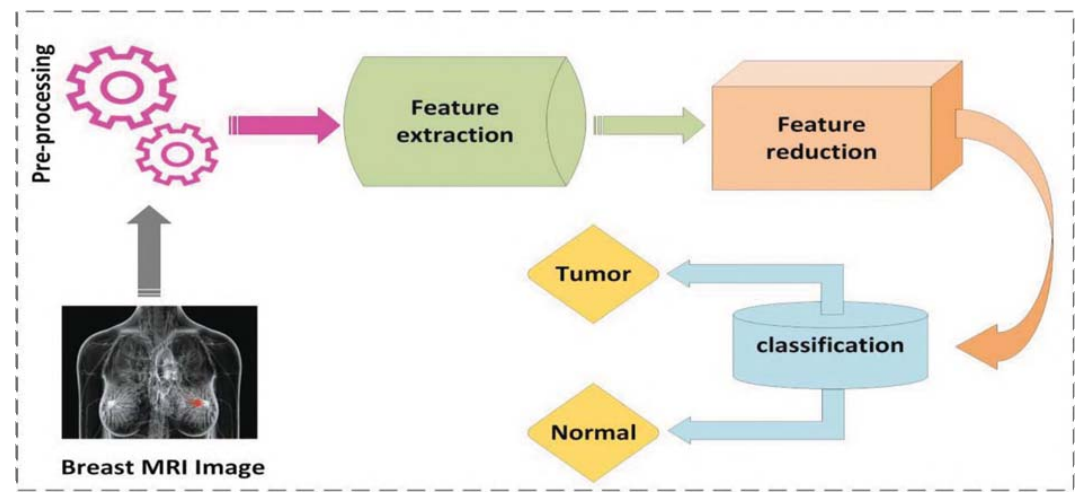
Automatic mri breast tumor detection using discrete wavelet transform and support vector machines
The human right is to live a healthy life free of serious diseases. Cancer is the most serious disease facing humans and possibly leading to death. So, a definitive solution must be done to these diseases, to eliminate them and also to protect humans from them. Breast cancer is considered being one of the dangerous types of cancers that face women in particular. Early examination should be done periodically and the diagnosis must be more sensitive and effective to preserve the women lives. There are various types of breast cancer images but magnetic resonance imaging (MRI) has become one of
Classifying Upper Limb Activities Using Deep Neural Networks
This paper presents a classification method using Inertial Measurement Unit (IMU) in order to classify six human upper limb activities. The study was also carried out to investigate whether theses activities are being performed normally or abnormally using two different neural networks: Artificial neural network (ANN) and convolutional neural network (CNN). Human activities that were included in the study: arm flexion and extension, arm pronation and supination, shoulder internal and external rotations. Before activities categorization, training data was obtained by the means of an IMU sensor
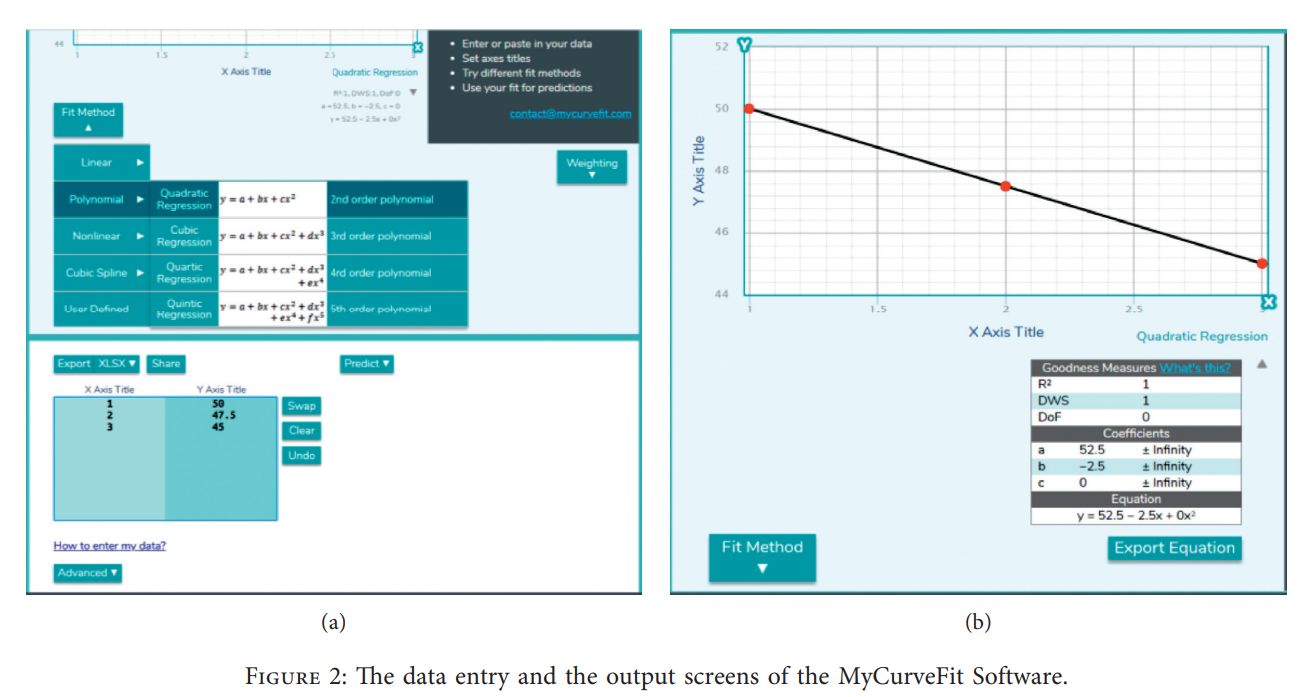
Optimum Scheduling the Electric Distribution Substations with a Case Study: An Integer Gaining-Sharing Knowledge-Based Metaheuristic Algorithm
This work is dedicated to the economic scheduling of the required electric stations in the upcoming 10-year long-term plan. The calculation of the required electric stations is carried out by estimating the yearly consumption of electricity over a long-time plan and then determining the required number of stations. The aim is to minimize the total establishing and operating costs of the stations based on a mathematical programming model with nonlinear objective function and integer decision variables. The introduced model is applied for a real practical case study to conclude the number of
Comparative Studies of Using Nano Zerovalent Iron, Activated Carbon, and Green Synthesized Nano Zerovalent Iron for Textile Wastewater Color Removal Using Artificial Intelligence, Regression Analysis, Adsorption Isotherm, and Kinetic Studies
Daily, a big extent of colored, partially treated textile effluents drained into the sanitation systems causing serious environmental concerns. Therefore, the decolorization treatment process of wastewater is crucial to improve effluent quality. In the present study, 3 different sorbent materials, nano zerovalent iron (nZVI), activated carbon (AC), and green-synthesized nano zerovalent iron (GT-nZVI), have been prepared for raw textile wastewater decolourization. The prepared nanomaterials were characterized via X-ray diffraction (XRD) spectroscopy, scanning electron microscopy (SEM), energy
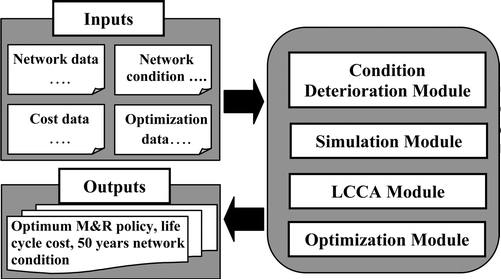
Multiobjective optimisation algorithm for sewer network rehabilitation
Understanding of deterioration mechanisms in sewers helps asset managers in developing prediction models for estimating whether or not sewer collapse is likely. Effective utilisation of deterioration prediction models along with the development and use of life cycle maintenance cost analysis contribute to reducing operation and maintenance costs in sewer systems. This article presents a model for life-cycle maintenance planning of deteriorating sewer network as a multi-objective optimisation problem that treats the sewer network condition and service life as well as life-cycle maintenance cost
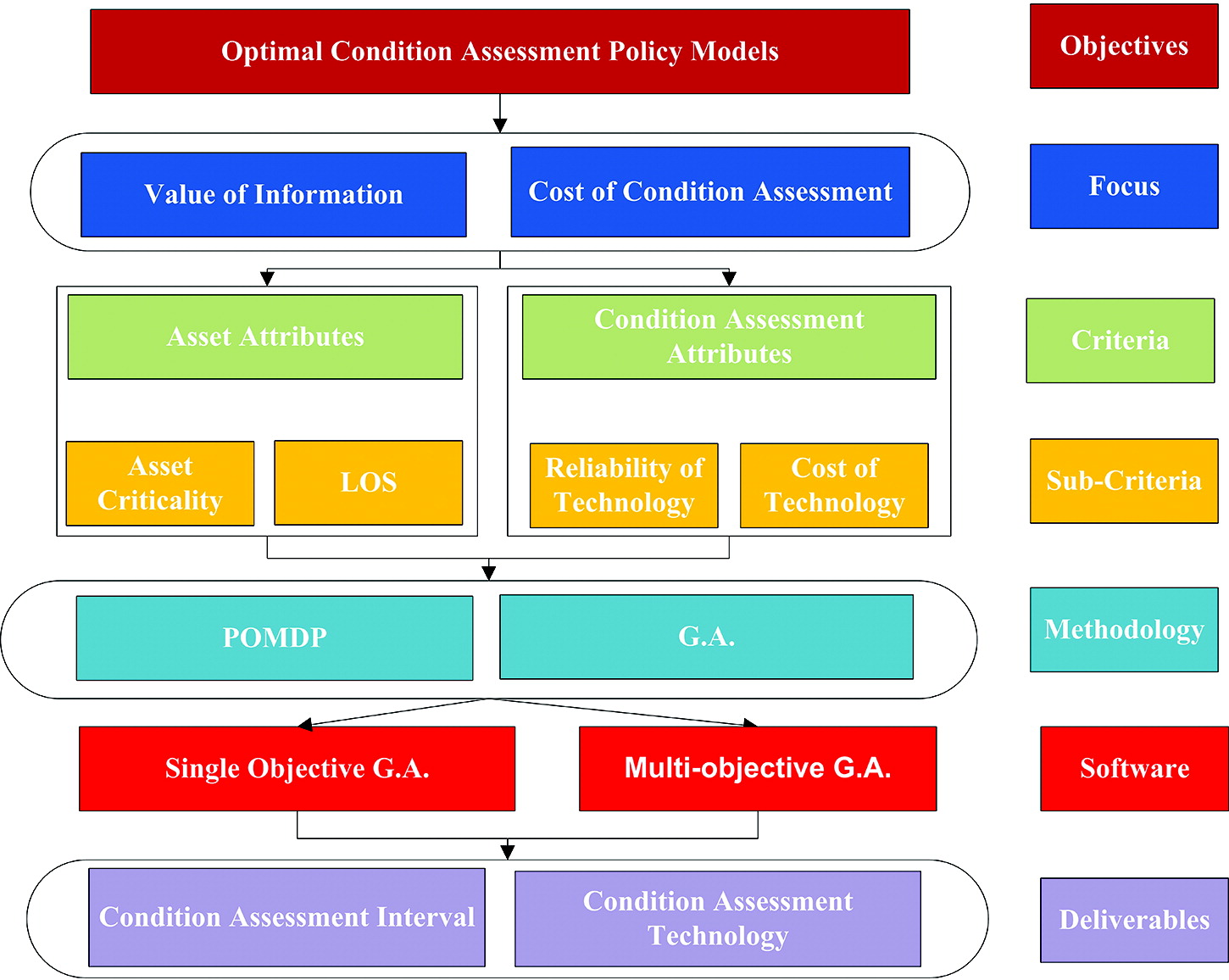
Multiobjective genetic algorithm to allocate budgetary resources for condition assessment of water and sewer networks
This paper presents a framework for optimizing condition assessment policies by balancing the revealed value of information with the cost of obtaining such information. The computational platform is based on augmenting the asset condition state with an expected level of accuracy. Inaccuracies due to condition assessment reliability are evaluated using the partially observable Markov decision process. The single objective genetic algorithm is used to select the most cost-effective assets to assess considering information inaccuracy under a fixed budget. The model is extended using