Faculty Office Ext.
1754
Faculty Building
UB1
Office Number
210
Dr. Mustafa Elattar, born in Cairo, Egypt in 1986, is a highly accomplished professional in the fields of biomedical engineering, image analysis, medical imaging, and artificial intelligence. He embarked on his academic journey at Cairo University, where he earned his bachelor’s degree in systems and biomedical engineering in 2008, Continuing his pursuit of knowledge and innovation, Mustafa received his Ph.D. in Biomedical Engineering and Physics, Faculty of Medicine, in 2016, from the Academic Medical Center, University of Amsterdam, The Netherlands. His doctoral research centered around developing a preoperative planning framework for transcatheter aortic valve implantation, showcasing his proficiency in leveraging advanced technologies to enhance surgical procedures. After completing his Ph.D., Mustafa joined the Netherlands Cancer Institute (NKI) as a postdoctoral fellow in 2016. During his time there, he focused on conducting research for image-guided radiotherapy, further expanding his expertise in the intersection of medical imaging and cancer treatment. In August 2017, Mustafa joined Nile University as an assistant professor at the Information Technology and Computer Science School. He is also the director of the Artificial Intelligence undergraduate program. Mustafa has gained valuable industry experience. He has worked in the research and development divisions of renowned companies such as Diagnosoft Inc., 3mensio B.V., PieMedical N.V., and Myocardial Solutions Inc. Furthermore, in August 2018, Mustafa founded Intixel Co. S.A.E., where he currently serves as its CEO
- Initiated the first African network for AI and Medical imaging enthusiasts, researchers and scientists.
- IVLP Impact Award from U.S. Department of State (2022).
- Best poster award at the Novel Intelligent and Leading Emerging Sciences Conference (2019).
- Top 5 startups in Young Business Hub Entrepreneurship Investment Summit, Bahrain (2019).
- Fareed Bader Award in World Entrepreneurs and Investments Forum (WEIF) (2019).
- Pitch deck winner and winning the best Health-tech startup at Takeoff Istanbul International Startup Summit after being evaluated by the jury members and 150+ mentors (2019).
- Top 10 Startups to be selected for the “2WiN Mentoring Program” supported by the German Chamber of Commerce (2019).
- Best poster in the Postgraduate Research Forum, Nile University (2018).
- Best Support for research assistant from Nile University (2018).
- Best Support for research assistant from Banque Misr (2017).
- 3rd place in Left ventricular segmentation challenge from cardiac MRI (STACOM 2011).
- Best poster in Image Analysis and Recognition Conference (2010).
- Full scholarship for master’s studies at Nile University (2008).
- Fourth Place in Made in Egypt (MIE) competition for the best graduation project (2007).
Pagination
- Medical Imaging
- Artificial Intelligence
- Image Analysis
- Knowledge Aggregation
- Graph Optimization
- Clinical Research
- Computational Cardiology
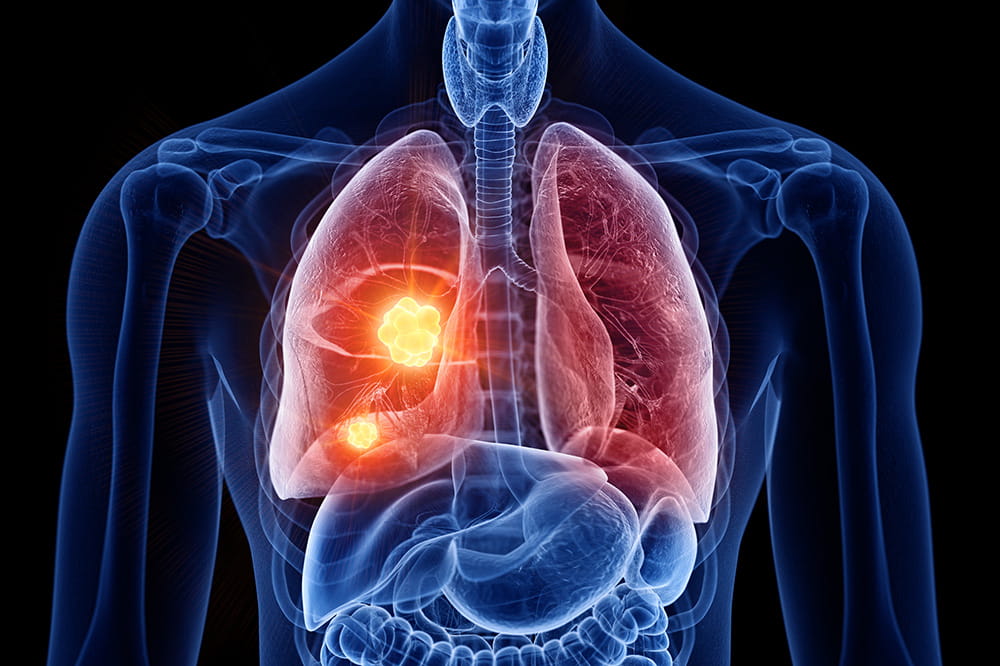
Lung Cancer Detection in Chest X-Ray Images Empowered by 3D Computed Tomography Deep Convolutional Radiomics (CXRClear)
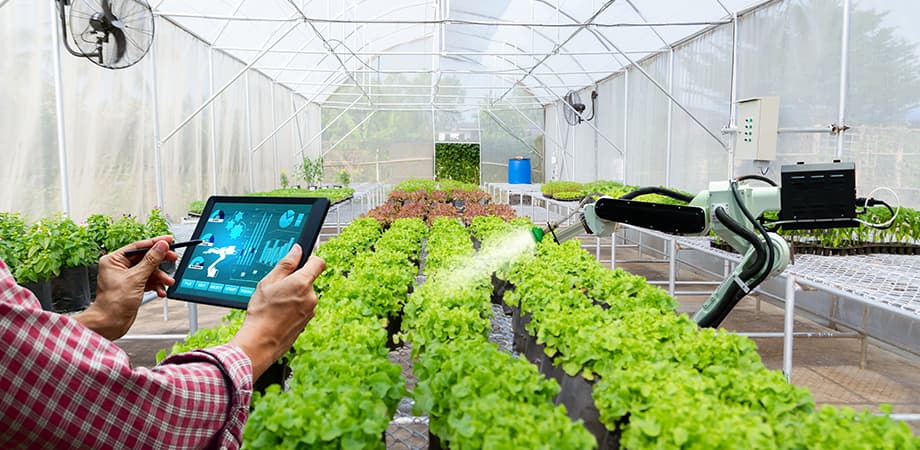
Smart Agricultural Clinic: Egyptian Farmer Electronic Platform for the Future
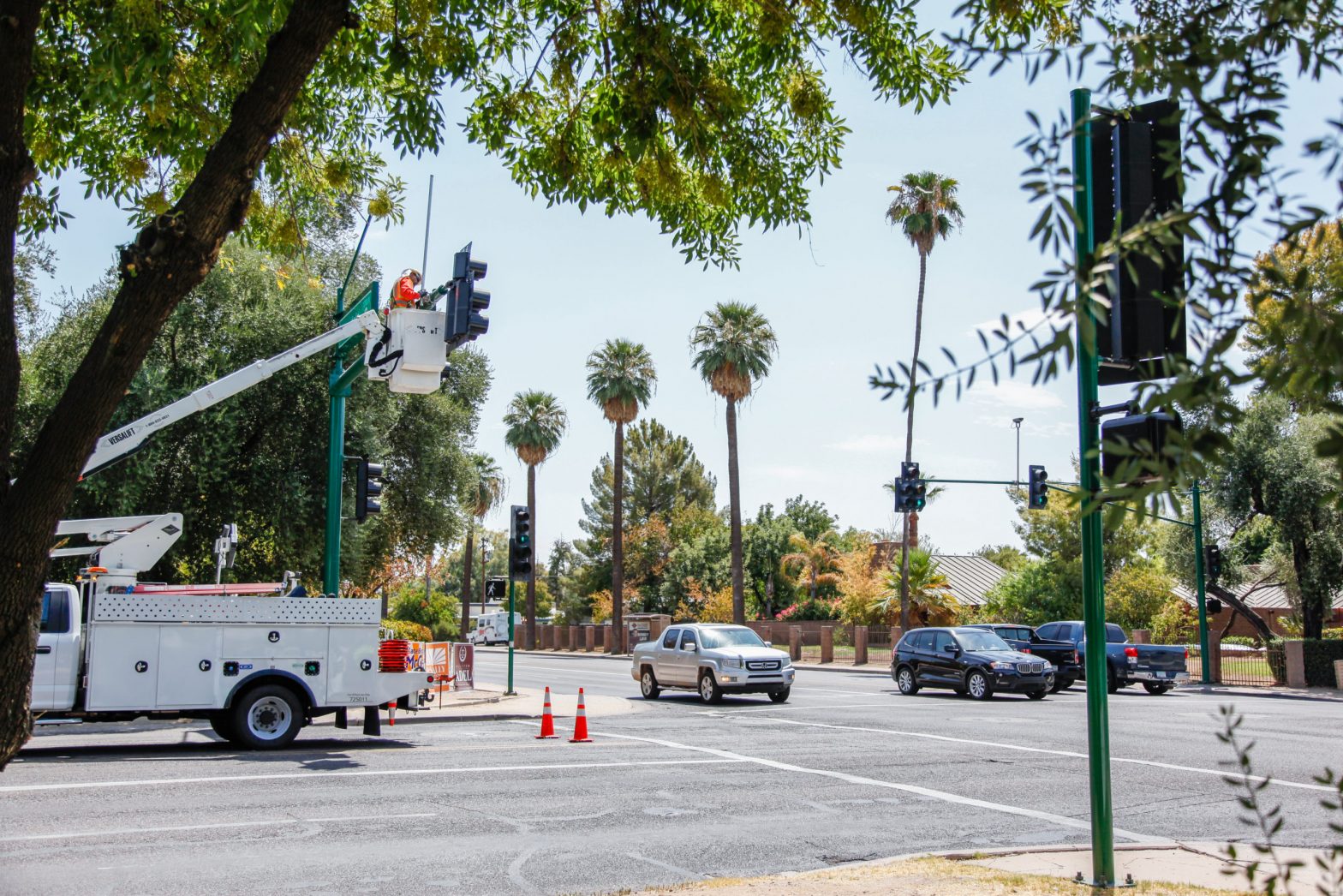