
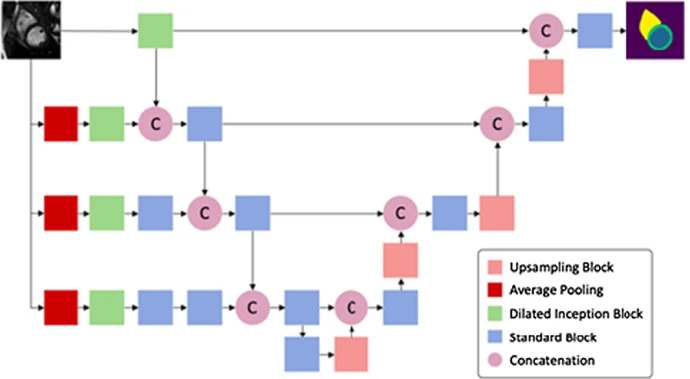
Multi-center, Multi-vendor, and Multi-disease Cardiac Image Segmentation Using Scale-Independent Multi-gate UNET
Heart segmentation in Cardiac MRI images is a fundamental step to quantify myocardium global function. In this paper, we introduce a pipeline for heart localization and segmentation that is fast and robust even in the apical slices that have small myocardium. Also, we propose an enhancement to the popular U-Net architecture for segmentation. The proposed method utilizes the aggregation of different feature scales from the image by using the inception block along with the multi-gate block that propagates the multi-scale context of the supplied data where the heart is subject to changes in scale, contrast and resolution due to the different imaging parameters from different vendor images. The model is trained using the data provided in Multi-center, Multi-vendor, and Multi-Disease Cardiac Image Segmentation Challenge (M&Ms challenge) with no prior training or transfer learning. The model achieved an average dice of 90%,84.5%, and 82.5% for the left ventricle blood pool, right ventricle, and Myocardium respectively. Moreover, the proposed pipeline took an average inference time of 0.0022 s per image when running on NVIDIA GTX 1080 TI GPU. © 2021, Springer Nature Switzerland AG.