
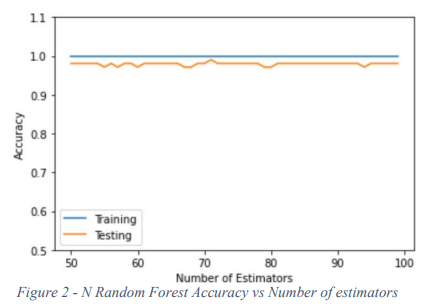
Diabetes Prediction Using Machine Learning: A Comparative Study
Diabetes is a common, metabolic disease, that results in a high level of blood sugar. Patients diagnosed with diabetes suffer from a body that cannot effectively use the insulin or cannot produce a sufficient amount of insulin. Providing a method of detection via symptoms that can be noticed by the patient can prompt the patient to seek medical assistance more promptly and in turn to be correctly diagnosed and treated. This paper proposed a solution for the problem using machine learning techniques. We applied eight algorithms on a data set of 521 subjects. The results are compared to each other to find the best algorithm for this task. The algorithms used are from different families which are logistic regression, support vector machines-linear and nonlinear kernel, random forest, decision tree, adaptive boosting classifier, K-nearest neighbor, and naïve bayes. The results show a clear advantage of using Random Forest with an accuracy of 98% having used 80% of the dataset for training and 20% for testing. © 2021 IEEE.