
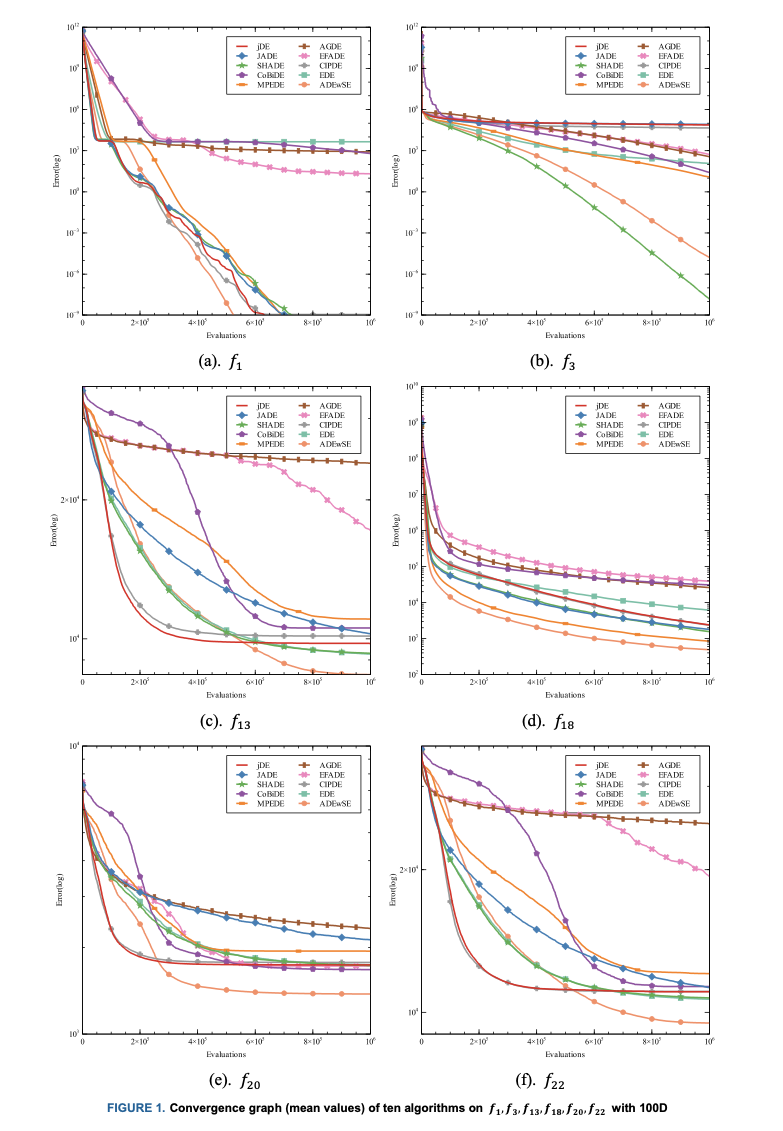
Adaptive differential evolution based on successful experience information
As a powerful optimization algorithm for solving nonlinear, complex and tough global optimization problems, differential evolution (DE) has been widely applied in various science and engineering fields. In this article, considering that the evolution direction of each individual is not fully exploited to guide the search process in most DE algorithms, a new DE variant ( named ADEwSE), which incorporates the successful experience of evolved individuals into classic ''current-to-pbest/1'' mutation strategy to reduce the randomness of search direction, is proposed. Moreover, crossover matrix sorting scheme based on real crossover rate, opposition learning of crossover rate and adaptive adjustment of top p% values are combined with the new mutation strategy to improve the global search ability. In addition, to improve the searching ability of ADEwSE further, an ADEwSE variant by introducing the linear reduction of population size is proposed. In order to verify and analyze the performance of ADEwSE, numerical experiments on a set of 29 test problems from CEC2017 benchmark for 30, 50 and 100 dimensions are executed. And the experimental results are compared with that of 21 state-of-art DE-based algorithms. Comparative analysis indicates that the ADEwSE and its improved version are competitive with these state-of-art DE variants in terms of solution quality obtained. © 2020 Institute of Electrical and Electronics Engineers Inc.. All rights reserved.