
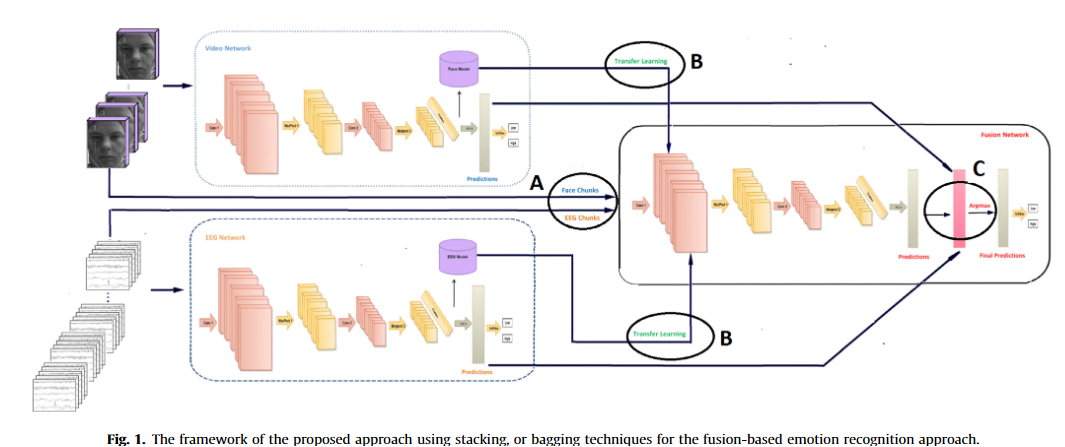
A 3D-convolutional neural network framework with ensemble learning techniques for multi-modal emotion recognition
Nowadays, human emotion recognition is a mandatory task for many human machine interaction fields. This paper proposes a novel multi-modal human emotion recognition framework. The proposed scheme utilizes first the 3D-Convolutional Neural Network (3D-CNN) deep learning architecture for extracting the spatio-temporal features from the electroencephalogram (EEG) signals, and the video data of human faces. Then, a combination of data augmentation, ensemble learning techniques is proposed to get the final fusion predictions. The fusion of the multi-modalities in the proposed scheme is carried out using data, and score fusion methods. Hence, three human recognition approaches are built to achieve the proposed goal. They are namely EEG-based emotion recognition approach, face-based emotion recognition approach, and fusion-based emotion recognition approach. For the EEG approach, the 3D-CNN is used to get the final predictions of the EEG signal. For the face approach, the Mask-RCNN object detection technique in combination with OpenCV libraries are first utilized to extract the exact face pixels with emotional content. Then, the Support Vector Machine (SVM) classifier is utilized to classify the 3D-CNN output features of the face chunks. For the fusion-based emotion recognition approach, two fusion techniques are experimented; bagging, and stacking. It is found that the stacking technique gives the best accuracy, and achieves recognition accuracies 96.13%, and 96.79% for valence, and arousal classes respectively using the grid search ensemble learning technique due to transferring the weights from the EEG, and the face approaches to the fusion-based emotion recognition approach. The proposed approach outperforms recent works in multi-modal emotion recognition field. © 2021