
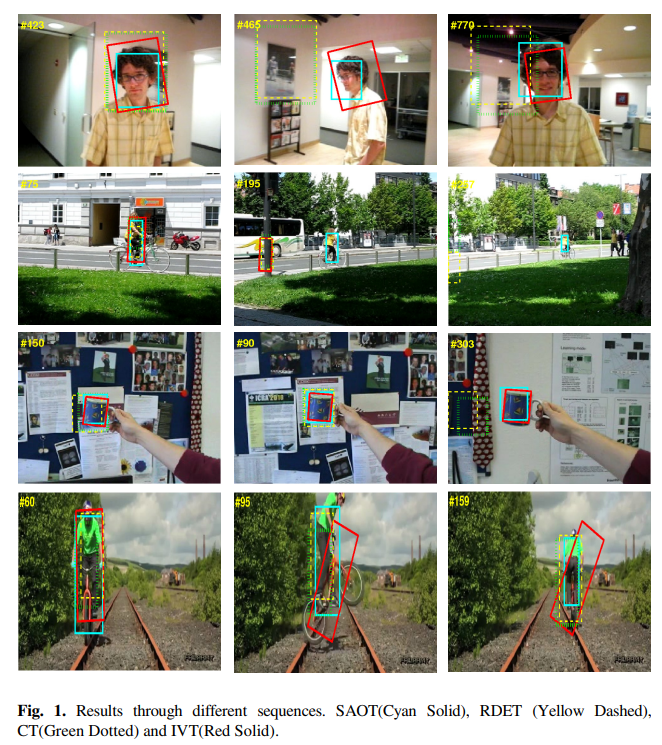
Scale-adaptive object tracking with diverse ensembles
Tracking by detection techniques have recently been gaining increased attention in visual object tracking due to their promising results in applications such as robotics, surveillance, traffic monitoring, to name a few. These techniques often employ semi-supervised appearance model where a set of samples are continuously extracted around the object to train a discriminant classifier between the object and the background whereas real-time performance is attained by using reduced object representations as in the case of the compressive tracking algorithm. However, because they rely on self updating, visual tracking algorithms are prone to visual drift especially when the object undergoes significant scale changes. In this paper, we present a real-time visual tracker that is adaptive to appearance and scale variations. The algorithm is divided into two phases: (1) object localization using a diverse ensemble of multiple random projections, and (2) scale estimation between an updated object template and the localized object position computed in the first phase. Experimental results obtained with publicly-available visual tracking datasets demonstrate that the proposed tracker provides robust tracking in case of significant scale variations with more accurate overlap and less visual drift. © Springer International Publishing Switzerland 2014.