
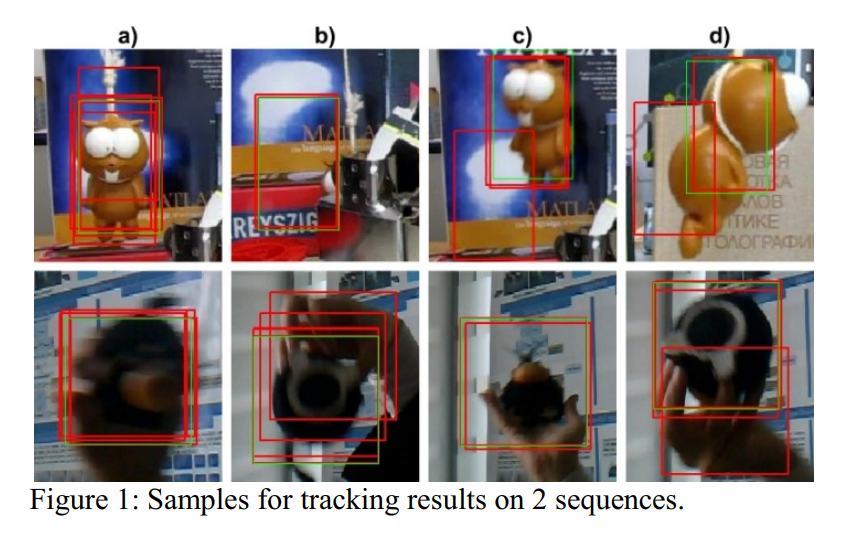
Robust real-time tracking with diverse ensembles and random projections
Tracking by detection techniques have recently been gaining popularity and showing promising results. They use samples classified in previous frames to detect an object in a new frame. However, because they rely on self updating, such techniques are prone to object drift. Multiple classifier systems can be used to improve the detection over that of a single classifier. However, such techniques can be slow as they combine information from different tracking methods. In this paper we propose a novel real-time ensemble approach to tracking by detection. We create a diverse ensemble using random projections to select strong and diverse sets of compressed features. We show that our proposed ensemble tracker significantly improves the accuracy of tracking while not using any additional information than that available to the single classifier, thus requiring little extra computational overhead. Our results also show that employing our multiple classifier system with feature subsets gives significantly better results than directly combining the features. © 2013 IEEE.