
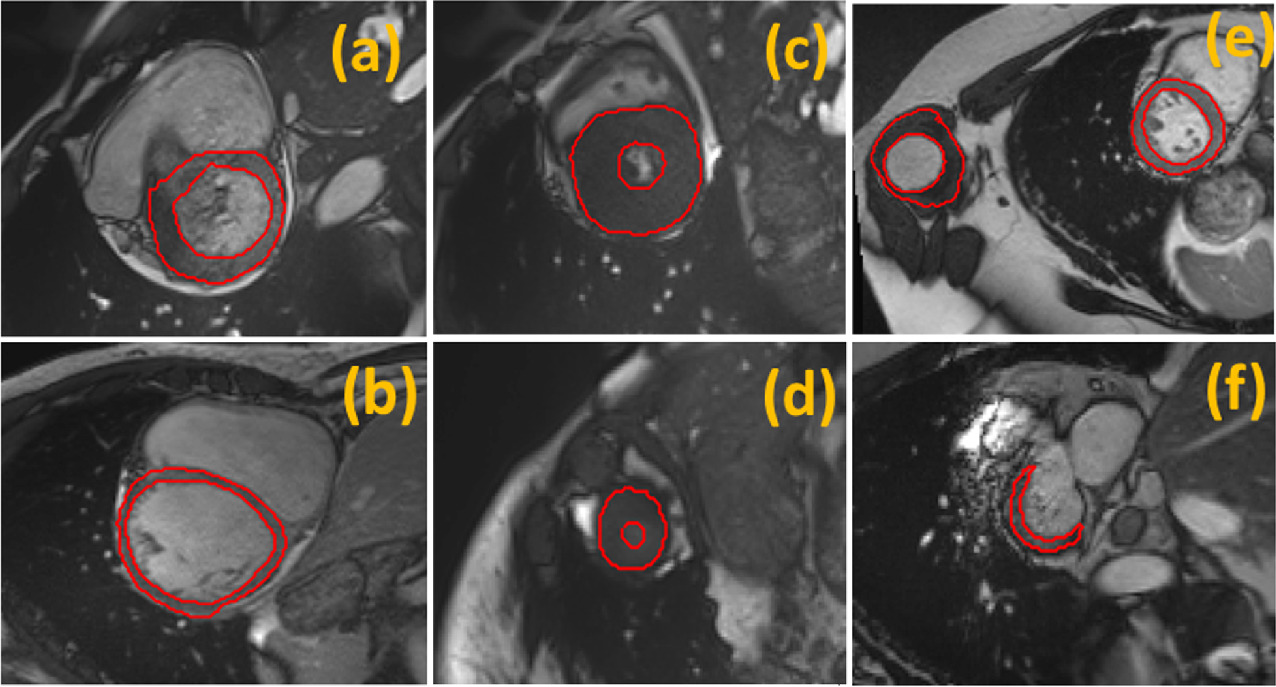
Left ventricle segmentation using scale-independent multi-gate unet in mri images
Left ventricle (LV) segmentation is crucial to assess left ventricle global function. U-Net; a Convolutional Neural Network (CNN); boosted the performance of many biomedical image segmentation tasks. In LV segmentation, U-Net suffered from accurately extracting small objects such as the apical short-axis slices. In this paper, we propose a fully automated left ventricle segmentation method for both short-axis and long-axis views. The proposed model utilizes U-Net architecture and Multi-Gate input block to enhance the performance by aggregating multi-scale features and adding different vision scopes providing a more robust model against scale variance of objects within the images. The proposed approach was validated against left ventricle segmentation challenge (LVSC) and Automated cardiac diagnosis challenge (ACDC). For LV myocardium segmentation, the proposed approach achieved mean dice index 82% on LVSC and 90% and 91% for end-diastole (ED) and end-systole (ES) time frames respectively on ACDC outperforming other published methods measurements at ED. For LV blood pool segmentation, mean dice index was 97% and 92.3% for the ED and ES time frames using ACDC, outperforming other methods’ ED measurements. © Springer Nature Switzerland AG 2020.