
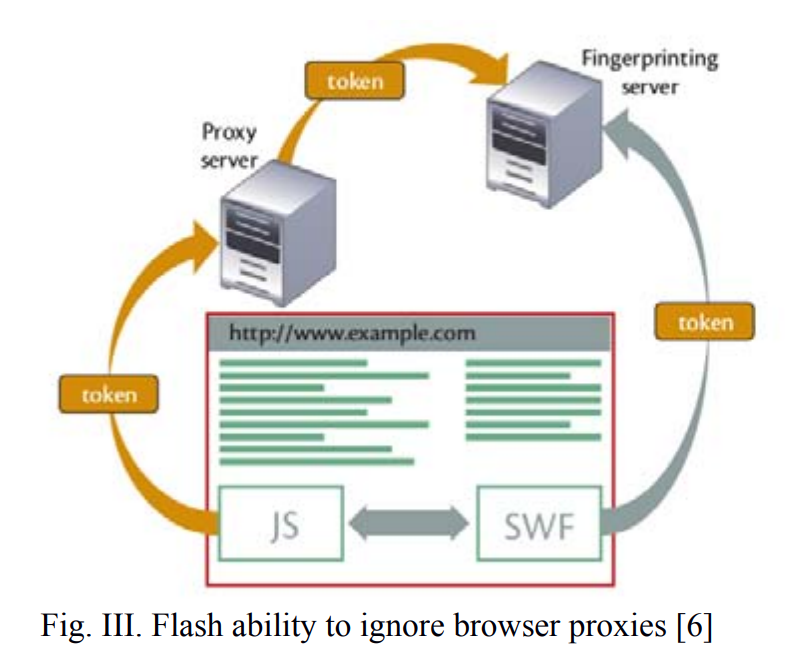
Decoding arm kinematics from EMG signals using Kalman filter
Myoelectric control of prosthetic arms provides a new hope for providing naturalistic movements to amputees. Extensive work has been made in recent years to use Electromyography (EMG) signals to enhance the operation of prosthetic arms. In this paper, we propose an EMG Kalman filter-based model, where we identify the relationship between the joint angles and recorded EMG signals. EMG signals were recorded from biceps and triceps muscles and used to train a Kalman filter decoder. We assessed the performance of the decoder by computing the correlation and the normalized root mean-square error (NRMSE) between the decoded and actual joint angles. When decoding using biceps EMG only, an average correlation of 0.61 was obtained with a NRMSE of 0.35. For triceps EMG only, an average correlation of 0.5 was obtained with a NRMSE of 0.5. Finally, when decoding the EMG of both the biceps and triceps muscles, the average correlation increased to 0.87 while the average NRMSE decreased to 0.18. These results outperform recent studies in similar applications which indicates the efficacy of the proposed decoder in decoding joint angles from recorded EMG. This could help in enhancing the control of prosthetic arms. © 2018 Association for Computing Machinery.