
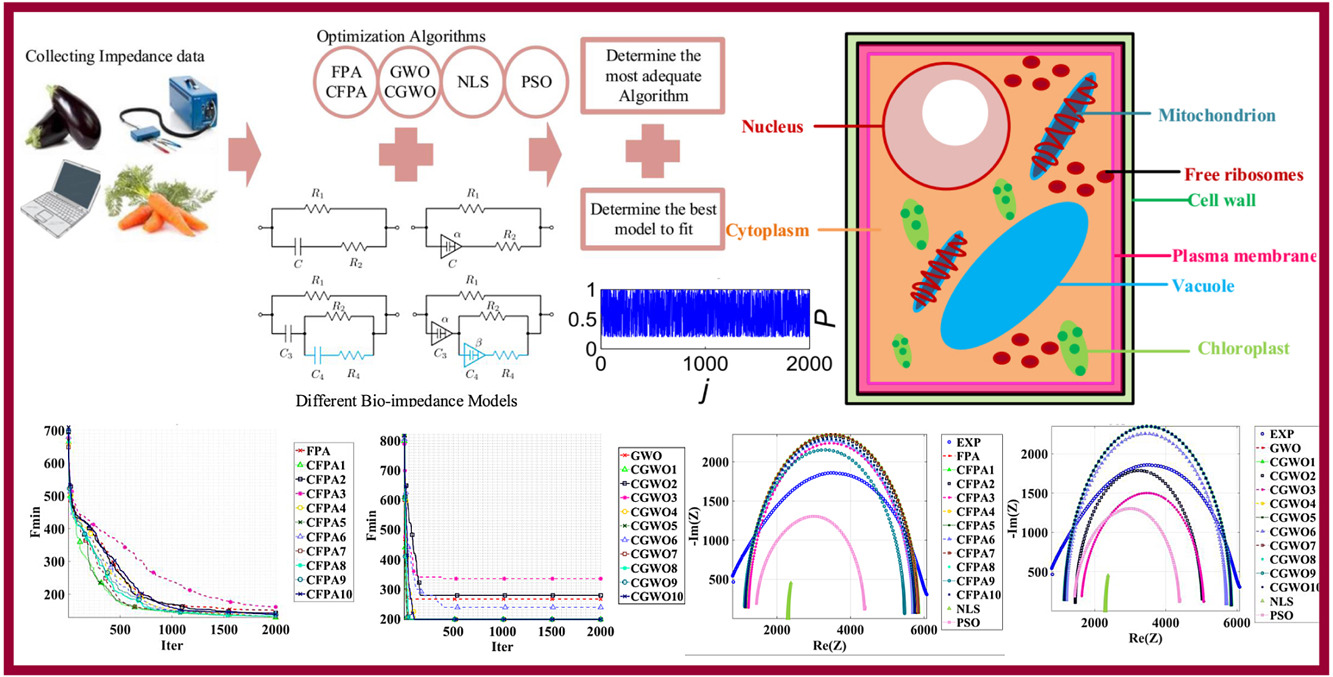
Chaotic Flower Pollination and Grey Wolf Algorithms for parameter extraction of bio-impedance models
Precise parameter extraction of the bio-impedance models from the measured data is an important factor to evaluate the physiological changes of plant tissues. Traditional techniques employed in the literature for this problem are not robust which reflects on their accuracy. In this paper, the Flower Pollination Algorithm (FPA), the Grey Wolf Optimizer (GWO) and ten of their chaotic variants are employed to extract the parameters of bio-impedance models. These models are the simplified Hayden and Double-Shell models in their integer and fractional order forms. Experimental data sets of three different vegetables are employed and the performance of the chaotic variants of FPA and GWO are tested mathematically over CEC 2017 benchmark functions. The optimization results are compared based on fitting error and execution time to visualize the trade-off between accuracy, consistency, and speed of convergence. The fitting results are compared with the traditional Non-Linear Least Square (NLLS) fitting and Particle Swarm Optimization (PSO) algorithms. The target is to select the most adequate algorithm for extracting the parameters for each presented model. For the simplified Hayden model, both CGWO and CFPA achieved good results. However, there is a trade off between time and consistency of the results. If the time factor (consistency of the results) is the dominant factor, the CGWO (CFPA) algorithm will perform better. For the double shell model, the GWO and CGWO achieve more accurate and more consistent results and less execution time than the FPA and CFPA algorithms. The fractional order version of the studied models provid a better fitting to the measured data compared with their integer order counterparts. © 2018 Elsevier B.V.