
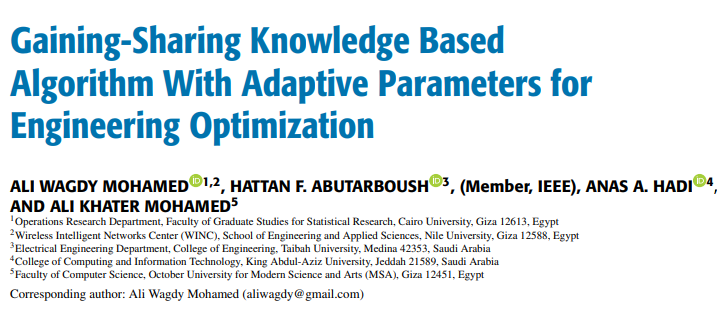
Gaining-Sharing Knowledge Based Algorithm with Adaptive Parameters for Engineering Optimization
As optimization algorithms have a great power to solve nonlinear, complex, and hard optimization problems, nature-inspired algorithms have been applied extensively in distinct fields in order to solve real life optimization cases. In this paper, modifications for the recently proposed Gaining-Sharing-Knowledge based algorithm (GSK) are presented for enhancing its performance. Gaining-Sharing-Knowledge algorithm is considered as a perfect example of modern nature-inspired algorithm that considered the human life behavior as a source of inspiration in order to solve optimization problems. GSK algorithm mimics the naturalistic phenomenon of gaining knowledge and sharing knowledge back in human being through two major phasis: junior phase and senior phase. The junior phase is based on generating a set of initial solutions (juniors) to start the search process. Subsequently, all individuals move to the senior phase through the interaction with the surrounding environment and the cooperation with other individuals throughout the search process. The main idea in this presented work is extending and improving the original GSK algorithm through proposing a new idea for adaptively obtain the values for the two significant control parameters in GSK: the knowledge factor and the knowledge ratio. Those two significant parameters have the responsibility of controlling the gaining and sharing knowledge during the junior and senior phases throughout the optimization process. Moreover, an adaptive scheme is presented to control the knowledge rate in order to simulate the gaining and sharing experience throughout the human being life span for a specific population by taking into consideration the diverse nature of any population. a non-linear reduction for the population size is used to improve the GSK algorithm's performance. Finally, a larger initial value of the initial population size (NP) for all dimensions are applied. The presented algorithm (APGSK) is tested on a very recent benchmark testbed on bound constrained numerical optimization that composed of distinct challenging optimization problems with different dimensions. The presented algorithm (APGSK) showed a superior performance comparing with the original GSK and with other four state-of-the-arts algorithms and the winners of CEC2020 competition. © 2013 IEEE.