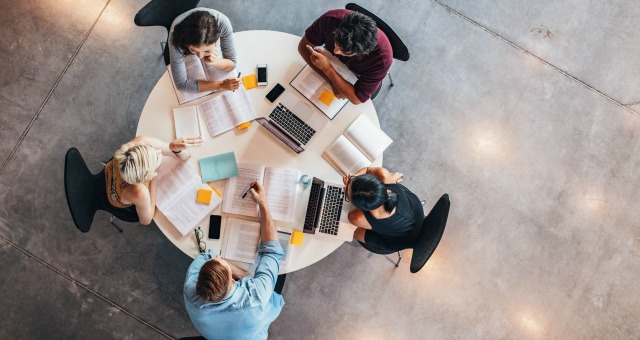
Novel Concept-based Image Captioning Models using LSTM and Multi-Encoder Transformer Architecture
Abstract
Captioning images involves using vision and language models to describe images concisely. Successful captioning requires extracting key information, including the image's topic. While state-of-the-art methods use topic modeling on caption text, this lacks consideration of the image's semantic information. Concept modeling, which extracts concepts directly from images and caption text, can better capture the image context and produce more accurate descriptions. This paper proposes novel image captioning models utilizing concept modeling, including an LSTM-based decoder and a novel multi-encoder transformer architecture. Evaluated on the Microsoft COCO dataset, the proposed models enhance image captions compared to state-of-the-art approaches, with reduced computational complexity.
Partners
Prof. Reda AbdelWahab, Prof. Khaled Mostafa, Prof. Mona Soliman, Dr. Asmaa Ahmed Othman