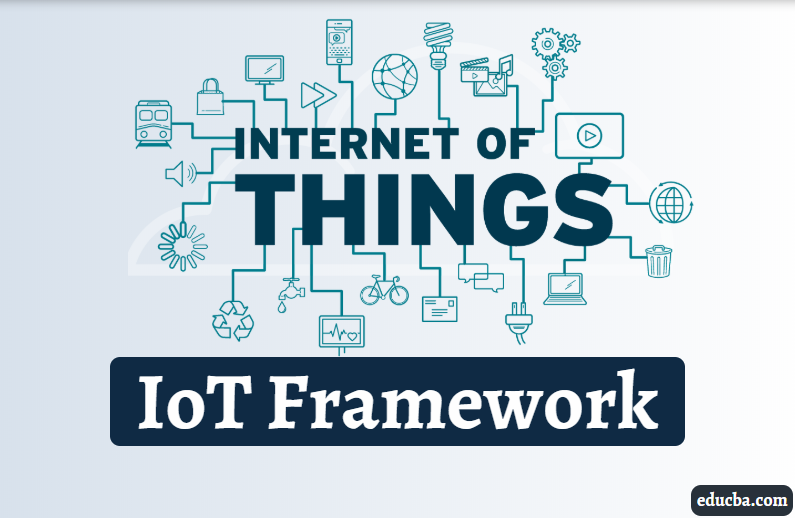
Low-Power Artificial Intelligence Framework for Internet of Things Applications
The Internet of Things (IoT) is penetrating many facets of our daily life with the proliferation of intelligent services and applications empowered by artificial intelligence (AI). Due to the massive scalability of modern IoT networks and increased data privacy, traditional AI algorithms require centralized data collecting and processing, which may not be practical in real-world application scenarios. Low-power AI, also known as federated learning (FL), is a distributed collaborative AI approach that can enable many intelligent IoT applications by allowing AI training at the IoT devices without data sharing. This project proposes a prototype solution integrated with a low-power AI (federated learning) framework that can offer better battery life and privacy preservation for different IoT applications.
Objective/Contributions:
- An in-depth understanding of the current low-power AI-based solutions for IoT applications.
- Develop a low-power AI framework that can meet the power and latency requirements.
- A prototype solution integrated with the low-power AI framework that helps in enhancing performance capabilities.
- Three high-quality scientific articles are to be published.
Outcome Publications:
- Samah Mohamed, Mahmoud Elbayoumi, Retaj Yousri, Ahmed Soltan, and M. Saeed Darweesh, “An Efficient DMO Task Scheduling Technique for Wearable Biomedical Devices,” in the 34th IEEE International Conference on Microelectronics (ICM 2022), Casablanca, Morocco, 2022. In Press.
- Retaj Yousri, Mahmoud Elbayoumi, M. Saeed Darweesh, Ahmed Soltan, and Ali Wagdy Mohamed, “Energy Aware Tikhonov-Regularized FPA Technique for Task Scheduling in Wearable Biomedical Devices,” In: Mohamed, A.W., Oliva, D., Suganthan, P.N. (eds) Handbook of Nature-Inspired Optimization Algorithms: The State of the Art. Studies in Systems, Decision and Control, vol 213. Springer, Cham. 2022. https://doi.org/10.1007/978-3-031-07516-2_8
- Retaj Yousri, Mahmoud Elbayoumi, Ahmed Moawad, M. Saeed Darweesh, and Ahmed Soltan, “A Novel Power-Aware Task Scheduling for Energy Harvesting-Based Wearable Biomedical Devices Using FPA,” in the 33rd IEEE International Conference on Microelectronics (ICM 2021), Cairo, Egypt, 2021.