
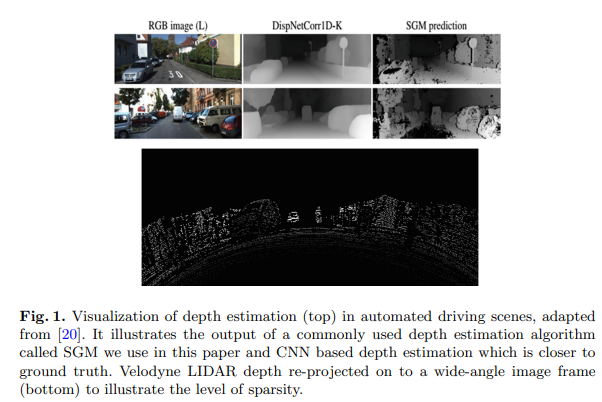
Depth Augmented Semantic Segmentation Networks for Automated Driving
In this paper, we explore the augmentation of depth maps to improve the performance of semantic segmentation motivated by the geometric structure in automotive scenes. Typically depth is already computed in an automotive system to localize objects and path planning and thus can be leveraged for semantic segmentation. We construct two networks that serve as a baseline for comparison which are “RGB only” and “Depth only”, and we investigate the impact of fusion of both cues using another two networks which are “RGBD concat”, and “Two Stream RGB+D”. We evaluate these networks on two automotive datasets namely Virtual KITTI using synthetic depth and Cityscapes using a standard stereo depth estimation algorithm. Additionally, we evaluate our approach using monoDepth unsupervised estimator [10]. Two-stream architecture achieves the best results with an improvement of 5.7% IoU in Virtual KITTI and 1% IoU in Cityscapes. There is a large improvement for certain classes like trucks, building, van and cars which have an increase of 29%, 11%, 9% and 8% respectively in Virtual KITTI. Surprisingly, CNN model is able to produce good semantic segmentation from depth images only. The proposed network runs at 4 fps on TitanX GPU, Maxwell architecture. © Springer Nature Singapore Pte Ltd, 2019.