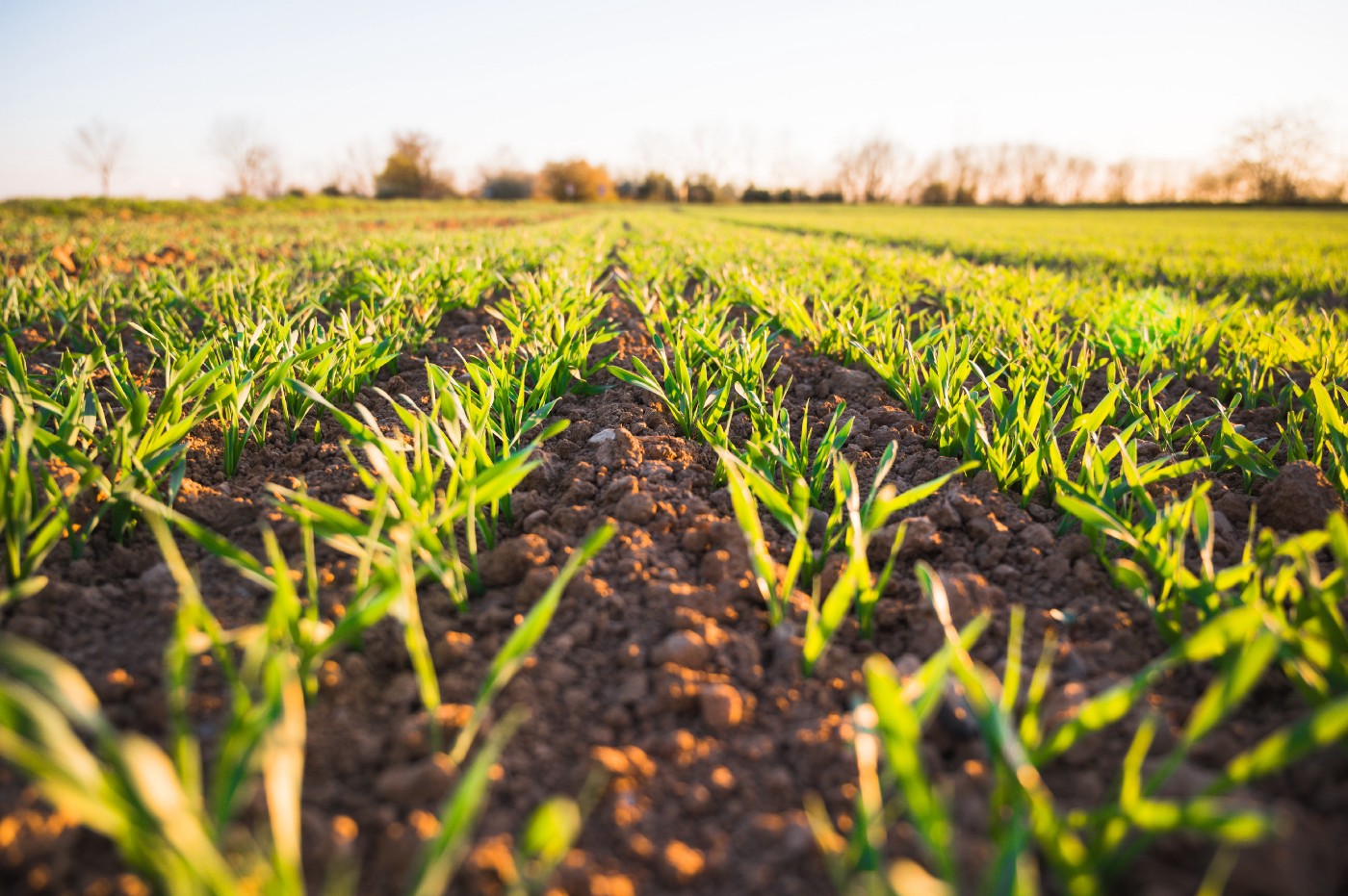
Rice Plant Disease Detection and Diagnosis Using Deep Convolutional Neural Networks and Hyperspectral Imaging
Objective/Contributions:
One of the main challenges of early detection of key rice blast disease is that it can be misclassified as the brown spot disease by less experienced agriculture extension officers (as both are fungal diseases and have similar appearances in their early stages) which can lead to wrong treatment. Given the current scarcity of experienced extension officers in the country, there is a pressing need and opportunity for utilizing recent technological advances in imaging modalities and computer vision/artificial intelligence to help in the early diagnosis of the rice blast disease. The former includes Hyperspectral Imaging (HSI) which has emerged as an effective modality to capture plant characteristics for precision agriculture and plant disease diagnosis applications. The latter comprises Deep Convolutional Neural Networks (DCNNs) that achieve state-of-the-art results in visual computing tasks especially for plant disease detection.
Although DCNN technology heavily depends on the availability of large datasets, there is a lack of public HSI datasets, especially for rice plant diseases. In this project, we propose a practical system for rice plant disease detection and diagnosis using DCNNS and HSI data. A novel DCNN architecture that utilizes a multi-stage fusion of HSI and RGB data will be used for accurate disease detection and diagnosis. In order to be able to train our DCNN model, we plan to collect a new HSI dataset for rice plant disease and also employ transfer learning techniques. The HSI dataset along with the DCNN architecture and analysis algorithms will be made publicly available to researchers and government agencies via an open-access portal to be hosted on Nile University servers.
Outcome: Publications
- Yara Ali Alnaggar, Ahmad Sebaq, Karim Amer, Elsayed Naeem and Mohamed ElHelw, “Rice Plant Disease Detection and Diagnosis using Deep Convolutional Neural Networks and Multispectral Imaging” , 11th International Conference on Model and Data Engineering, Egypt, 2022.
- The collected dataset contains 3815 samples and covers three classes: blast disease, brown spot disease and healthy leaves.
- Each sample is composed of a pair of images collected by the mobile camera (RGB) and MAPIR camera (R-G-NIR) simultaneously.